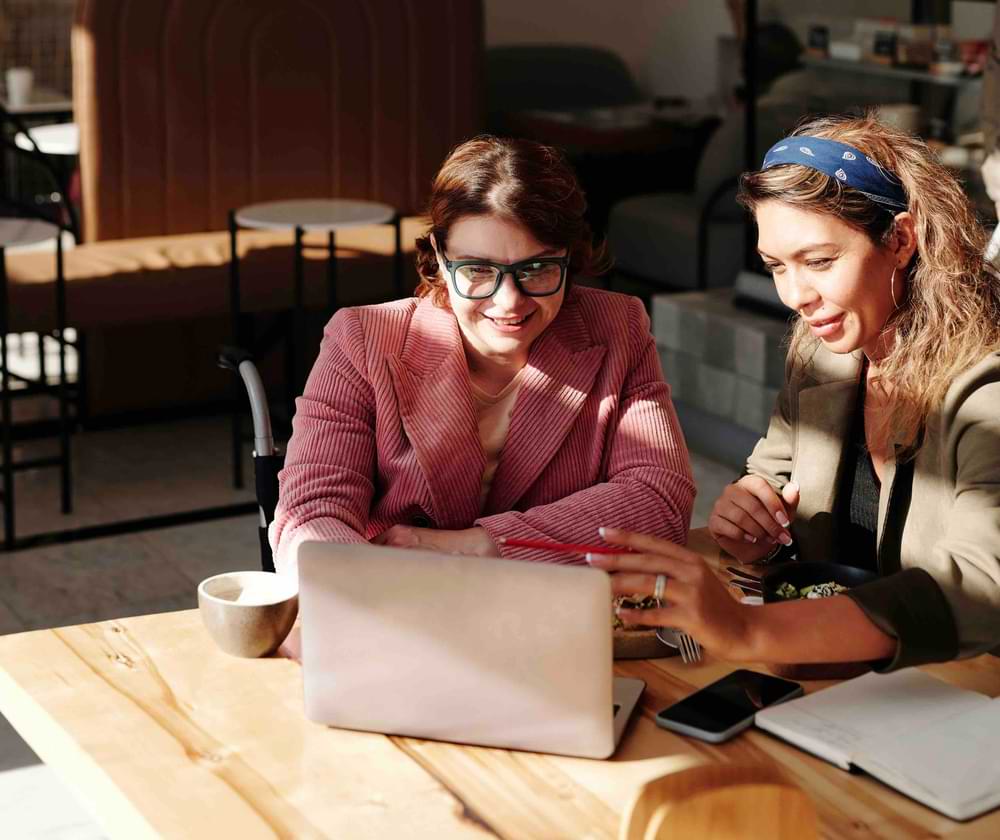
Open-Ended Questions: Tips & Tricks for Getting the Most Out of Your Survey
When writing a market research survey, it’s essential to think about what type of data you are ultimately looking to gather from your research. While most online surveys are great for collecting quantitative data, peppering in a few open-ended questions can provide you and your team with a qualitative perspective. By opening up your study to open-ended responses, you will provide more freedom for your respondents’ to give open and honest feedback.
But what are open-ended questions, and how can you write them effectively? In this article, we’ll explain what open-ended questions are, their benefits and limitations, and provide examples that will help you craft your open-ended questions.
-
- What are open-ended questions?
- What are closed-ended questions?
- What are some benefits of open-ended questions?
- What are some limitations of open-ended questions?
- Open-ended question examples
- What does PureSpectrum do to ensure high-quality open-ended research?
What are open-ended questions?
Open-ended questions are free-form survey questions that ask people to respond to a question in their own words. By leaving an answer field open, these questions are designed to elicit more information than is possible in multiple-choice or closed-ended survey question formats.
Open-ended questions are broad and have many possible answers. These exploratory questions allow researchers to learn more about their target markets by enabling them to answer questions in whatever words they choose. This freedom may offer data that was not expected and would otherwise not have been captured as a closed-ended answer.
Open-ended questions are also a great way to assess unaided brand awareness in brand trackers. By asking people to recall what brands they think of without a list to choose from, researchers can have an unbiased view of what brands a particular consumer has top of mind.
What are close-ended questions?
Close-ended questions pose a question to a respondent and then provide responses for the person to choose between. Closed-ended questions are the more common question type in online quantitative market research.
There are many closed-ended question types, such as multiple select, single select, true/false, ranking and heatmaps. These types of closed-ended questions are helpful because the data they provide is easy to analyze in crosstabs and compile into reports. Read Survey Methodology: What You Need to Know to Write Great Surveys to learn more about survey methodology.
Closed-ended questions are more straightforward for respondents to answer, they are often more mobile-friendly, and their replies don’t require as much thought as open-ended questions. Questionnaires that favor a majority of closed-ended questions tend to be shorter in length and have higher completion rates than surveys that ask many open-ended questions.
If adding opened questions to your survey is not possible because of industry limitations like healthcare adverse event processing requirements, researchers should add “other (specify)” to closed-ended answer lists. This answer gives the respondent a way to let surveyors know that they may not have captured all the feasible answers to a question.
What are the benefits of using open-ended questions?
Open-ended questions are beneficial because they allow respondents to answer a question in their own words. Some respondents react very favorably to being able to give reasoning behind their opinions. Survey participants may also favor open-ended questions because it feels more like a conversation than a basic survey.
Having respondent comments included in research reporting is very powerful and meaningful to many companies. The feedback collected in open-response questions provides executive teams with exact sentiments directly from their target customers and clients. These responses can also aid marketing teams with new ideas for campaigns and even provide new taglines.
Open-ended questions are evolving to also allow for video replies. This is a newer way to not just get feedback but to also understand the context and the emotions involved in a participant’s response. Video answers are another really powerful tool to use in stakeholder presentations.
A final benefit of open-ended questions is their ability to reduce response bias in a survey. Because this question type does not offer answer prompts, the answers collected have a greater chance of accurately representing what a participant knows or feels. Check out this guide to learn more about survey bias and ways to avoid it.
What are some limitations of open-ended questions in online surveys?
While some respondents love the ability to share their opinions in their own words, others may feel weighed down by crafting an answer from scratch. If an open-ended question is too long or complex, it may result in longer field times due to many respondents dropping out to avoid typing a response.
As previously discussed, open responses provide an opportunity to gain insight into varied opinions on a topic, but these questions can lack the statistical significance needed for conclusive research. Because each answer is unique, they are harder to quantify and perform data cuts on. However, there are ways that online market research platforms, like PureSpectrum, can help this data to be viewed more quantitatively.
The PureSpectrum team can perform coding so that statistical significance and various data cuts can be performed on open-ended data collected in a survey. This important service is offered on all PureSpectrum full-service studies. Open-ended data can be coded and integrated into final data files, banners, etc.
Another way to analyze open-ended responses is in a word cloud. This feature can be found in several online market research tools, including the PureSpectrum Insights Platform. A word cloud highlights words used repeatedly in open-ended feedback. Word clouds offer an easy way to visualize common answers that are given to open-ended questions. Below is an example from our EV Brand Tracking Survey.
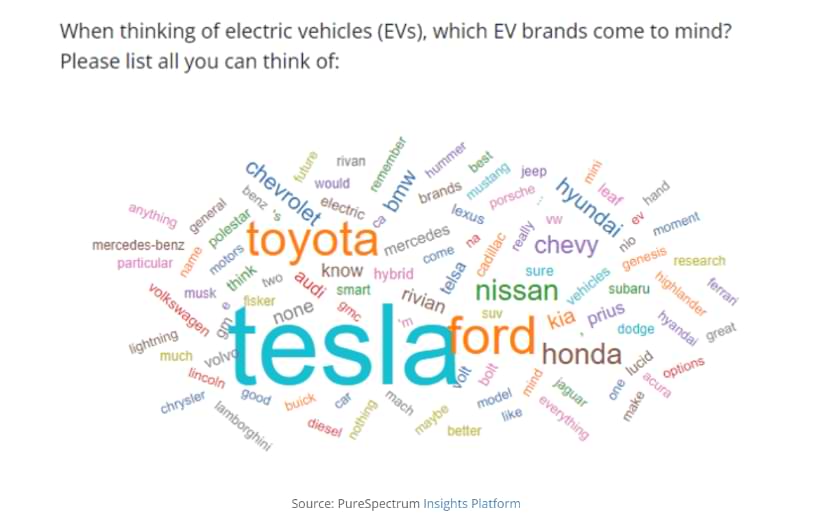
Open-Ended Question Examples
Open-ended questions can be about almost anything a researcher is looking to survey. Using open-ends in online market research surveys can effectively gather several types of qualitative insights.
1. Open-Ended Questions Measure Unaided Awareness
In online surveys, an optimal usage for open-ends is a way to check unaided awareness of a product or market segment. These types of open-ended questions aim to understand the respondent’s baseline knowledge. With these questions, you should ask the participant for the first idea or brand that comes to mind on your topic. Unaided awareness questions are often found in brand tracking surveys.
-
-
- When thinking of [CATEGORY], which brands come to mind? Please list all you can think of.
-
2. Understanding Positive or Negative Responses with Open-Ended Questions
Open-ends can also effectively understand why a survey participant has reacted positively or negatively to a concept or idea. A researcher may get more in-depth and truthful responses by asking them to write why they responded in a particular way. These types of questions are often found in concept tests. Check out our concept testing basics and FAQs to learn more about this surveying method.
-
-
- Earlier, you mentioned that you are likely to buy this [CONCEPT]. Why, specifically, are you likely to buy it? Please be specific.
- Earlier, you mentioned that you are not likely to buy this [CONCEPT]. Why, specifically, are you not likely to buy it? Please be specific.
-
3. Open-Ends to Collect Further Consumer Feedback
When looking for feedback from your target consumers, it can help to include an open-ended question that is completely open and not leading. In this way, companies learn there may be other important issues consumers are facing that the company did not realize. This may also give insight into other survey topics to explore next.
-
-
- What else would you like to tell us about [BRAND/PRODUCT]?
-
4. Open-Ends As a Data Quality Check
Asking a survey participant to answer general red-herring questions can be a strategic way to determine if a person is engaged in a survey, reading the questions thoroughly, and being able to answer correctly in the same language in which the survey is being fielded. Examples of data quality check questions are:
-
-
- How would you describe yourself in a short sentence?
-
While this question does not have exact right or wrong answers, it is easy to analyze if the respondent understood and attempted to answer the question posed correctly.
Open-ended questions are a great way to collect qualitative data in a quantitative way. By asking a few of these survey questions to your participants you can collect real responses to questions that may surprise you. Open-ended questions are a strong compliment to any survey that is primarily asking closed-ended questions.
While not all survey participants like being asked to write their answers, many do prefer to lend their own voice instead of being prompted at every response. When asking open-ended questions it’s important to remember to write them in a clear and concise manner so as to not lead the respondent in any specific manner. As an additional best practice, make sure all your survey questions are easily visible in a mobile setting as well as on a desktop.
What does PureSpectrum do to ensure high-quality open-ended research?
PureSpectrum is passionate about data quality. Alongside our award-winning data quality measure PureScore™, we now also offer Puretext™. Utilizing natural language processing technology, PureText™ reviews each open-end response sourced on PureSpectrum for curse words, gibberish, or low-quality answers. Respondents who do not accurately answer open-ended screening questions will have their PureScore negatively affected and be barred from entering surveys. Once in a study, open responses that don’t match quality standards are flagged for review, making it easier for researchers to remove the answers from their data set.
Are you ready to start a survey? Our experienced and dedicated services team is here to help you get the most out of your research budget. Reach out below!
About PureSpectrum
PureSpectrum offers a complete end-to-end market research and insights platform, helping insights professionals make decisions more efficiently, and faster than ever before. Awarded MR Supplier of the Year at the 2021 Marketing Research and Insight Excellence Awards, PureSpectrum is recognized for industry-leading data quality. PureSpectrum developed the respondent-level scoring system, PureScore™, and believes their continued success stems from their talent density and dedication to simplicity and quality.
In the few years since its inception, PureSpectrum has been named one of the Fastest Growing Companies in North America on Deloitte’s Fast 500 since 2020, and ranked for three years in a row on the GRIT Top 50 Most Innovative List and the Inc. 5000 lists.